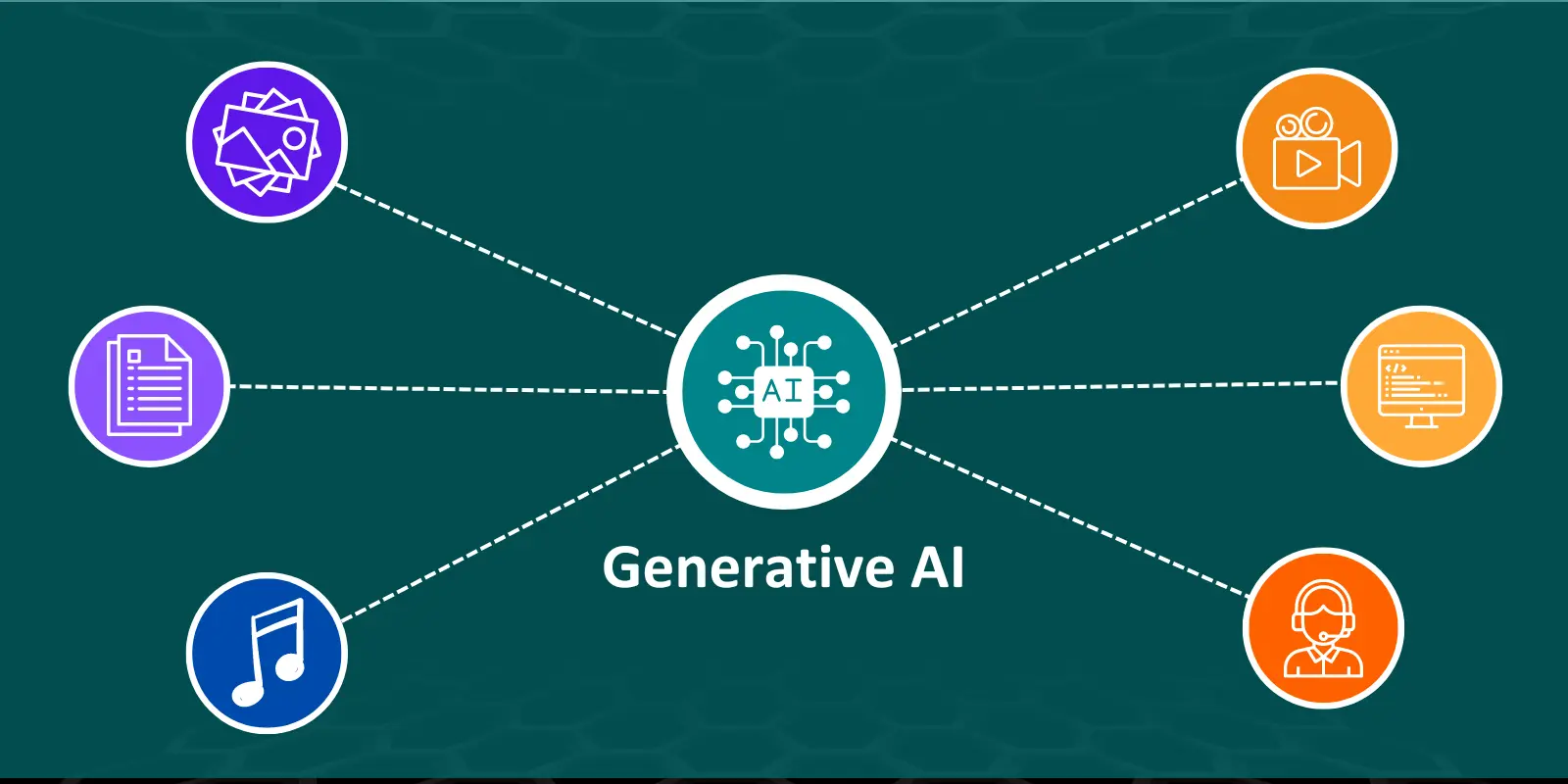
How Generative AI Works: Generative AI, at its core, focuses on creating new content – be it text, images, audio, or videos by learning patterns and structures from vast amounts of data. This remarkable technology is powered by advanced machine learning models and techniques, which enable it to understand, synthesize, and produce original outputs. Here’s an in-depth look at how generative AI works:
Foundation: Training the Model
Generative AI begins with a process called training, where the model learns from massive datasets. This step involves:
- Data Collection: Feeding the model with diverse information, such as text, images, or videos, depending on its purpose.
- Supervised or Unsupervised Learning: In supervised learning, the model learns from labeled data, while in unsupervised learning, it analyzes unstructured data to find patterns on its own.
- Neural Network Architecture: Complex structures like transformers (used in GPT models) or Generative Adversarial Networks (GANs) are employed to process and analyze data efficiently.
Key Techniques in Generative AI
Different methodologies are used to enable generative AI to produce content. Two prominent approaches are:
a. Generative Adversarial Networks (GANs)
GANs consist of two neural networks working together:
- Generator: Produces new content, such as images or videos.
- Discriminator: Evaluates the generated content to determine whether it’s real or fake. The generator and discriminator compete, gradually improving the quality of the output until it becomes indistinguishable from genuine data.
b. Transformers
Transformers are widely used in natural language processing models like GPT. These architectures rely on:
- Attention Mechanisms: Identifying key relationships between data points to generate coherent and contextually relevant content.
- Sequential Understanding: Processing input data (like text) in a structured order to produce logical outputs.
Content Creation Process
The steps for generating AI content include:
Step 1: Input
The user provides input, such as a prompt, query, or specific instructions. Examples could be:
- Text prompts like “Write a poem about the ocean.”
- Image descriptions like “Generate a painting of a sunset over a mountain.”
Step 2: Processing
The AI analyzes the input and determines what content to create. It uses:
- Pre-trained knowledge from its dataset.
- Contextual understanding to interpret meaning and requirements.
Step 3: Output Generation
The model generates the content by:
- Building text, images, or other outputs step by step.
- Applying learned patterns and creative elements.
Refinement and Feedback
Some generative AI systems allow users to refine the output:
- Interactive Adjustments: Modifying generated content based on user feedback.
- Fine-Tuning Models: Personalizing the model’s response to meet specific requirements.
Advantages of Generative AI
Generative AI excels in:
- Creativity: Producing content that feels imaginative and unique.
- Efficiency: Automating labor-intensive processes like writing, designing, and editing.
- Accessibility: Enabling users with minimal skills to create professional-quality outputs.
Challenges in Generative AI
Despite its benefits, generative AI faces some hurdles:
- Bias: Models can inherit biases from the data they’re trained on.
- Authenticity Concerns: Generated content might be mistaken for human-created work.
- Ethical Use: Ensuring responsible application to prevent misuse.
Conclusion
Generative AI combines cutting-edge algorithms, neural networks, and creative synthesis to bring imaginative ideas to life. As technology advances, its potential to revolutionize industries—from art and entertainment to healthcare and education—continues to grow. Whether generating lifelike images or composing original text, generative AI demonstrates the incredible power of artificial intelligence.